The Challenge of Performance Model Selection
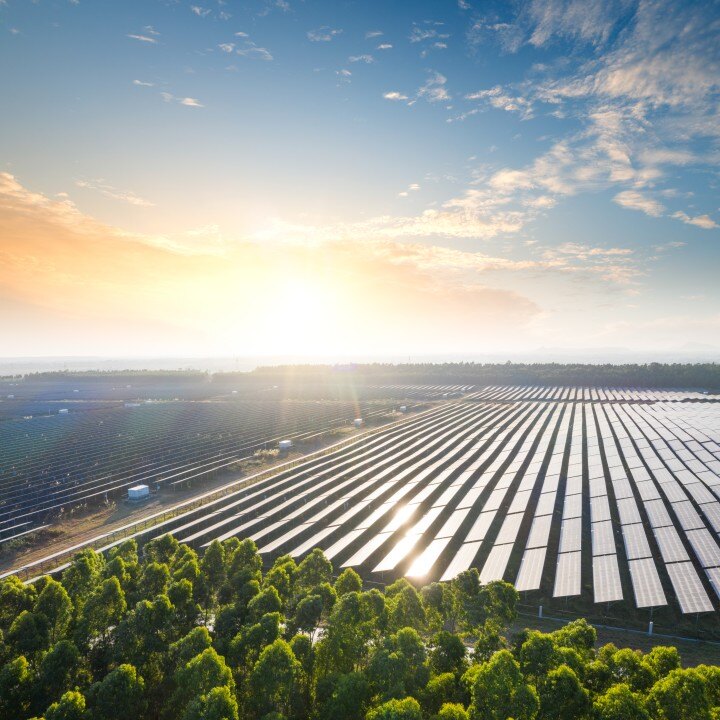
Stay in touch
Subscribe to our newsletter for expert insights, company updates, and the latest in renewable energy management solutions.
This is the fourth article in an eight-part series about the top four challenges in solar performance monitoring and how to overcome these challenges.
In the first three articles, we discussed the challenge of working with imperfect operating data, the challenge of scale and the challenge of data granularity. Most solar monitoring software platforms fail when attempting to estimate the performance of many small and un-instrumented assets based on imperfect, high-volume/velocity/variety data.
In this article, we discuss another common problem with solar monitoring systems: the challenge of selecting the right performance model.
DEFINING THE CHALLENGE OF SELECTING THE RIGHT MODEL
The challenge of selecting the right performance model when estimating the performance of an energy asset could be considered a good problem to have. When I first got into the power business over 38 years ago, the only choice available at the time was to build a first principles (physical) model of the plant and the equipment.
Physical models were usually an attempt by performance engineers to characterize the current state of the asset by calculating its theoretical optimal state, then using plant instrumentation to estimate losses. Asset performance was calculated by subtracting current losses from as-built capacity and efficiency specifications. The process was straightforward if the right sensors were installed and the asset was at a steady state during the evaluation period.
Today, a great variety of performance models exist in addition to physical models: machine learning, neural networks, artificial intelligence, statistical models and digital twins. The flip side of the benefit of model diversity is that with all these choices I now need to figure out which is the best model to apply at each step in my performance analysis data process.
Then, once I choose a model, I need to choose which sub-model is the right one for the performance evaluation task. For example, for PV plant capacity models we have the ASTM 1 model, the ASTM 4 model, the Perez model and many others to choose from. How do I know which one is the right one for each step in the flow of data through my performance analysis engine?
THE PROBLEM WE ARE TRYING TO SOLVE
Before we answer that question, let’s not forget the question we are attempting to answer. The purpose of an energy performance model is to create an estimate of the current capability of an asset. Performance engineers often call this the asset’s “expected production.”
Once I have an estimate of the asset’s expected production, I can compare that with how it is actually performing. The difference between actual and expected production is then calculated — the “residual.” The residual is then compared to a fixed or dynamic control limit. If the residual exceeds the control limit for a certain period of time, an event is triggered. The asset is considered to be “out of statistical control,” meaning its deviation from expected performance is large enough that I should be concerned about it.
For example, if the asset we are evaluating is an inverter, we measure its actual energy production over time with an electric meter and compare that value with its expected production for the same time period. If the inverter production residual exceeds our statistical control limit, we generate a notification and add some MWh to our inverter loss allocation bucket for that reporting period.
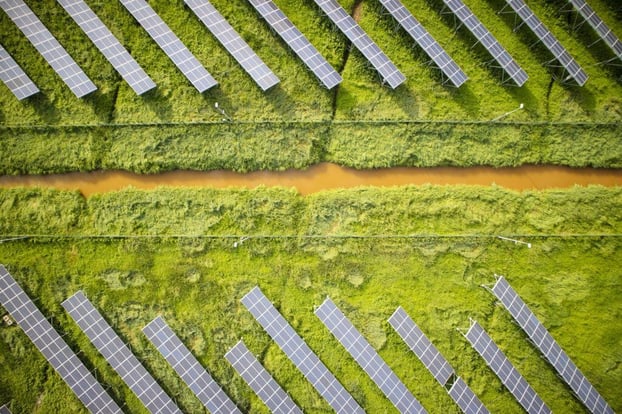
Are You Keeping Track of Supplemental Information?
This all sound easy enough. However, once you have determined the best model for each step in the performance engine data flow, you also need to keep track of a lot of additional supplemental information to make sure the performance model is aware of all of the factors that can have a material impact on its estimating ability.
For example, while we are estimating inverter expected production for that time period, our performance engine also needs to know:
- Were there any inverter clipping events?
- Were there any plant curtailment events?
- Were there any plant controller events?
- Were there any inverter outage events?
- Were there any inverter derating events?
- Were there any inverter comm losses events?
- Were there any sub-array events that would impact inverter production?
Each of these event types — and many more — must be accounted for when evaluating the expected performance of an inverter. If proper loss allocation is not performed, these underperformance events will be allocated to the inverter and the real source of the problem may go unnoticed.
One Model Does Not Fit All
Even with my simple example above, it should be clear that creating a scalable, robust and maintainable asset performance model for the solar power asset class is no easy task. As with many industry-specific problems, silver bullet solutions are few and far between. Selecting the right model to apply to the right asset at the right step in the performance analysis data flow takes deep domain expertise.
Subject Matter Expertise is Needed
When I advise people to consider investing in a scalable, robust and maintainable asset performance management system, they often ask, “Why can’t I just purchase the latest general purpose ML or AI tool, plumb it up to my operating data via Python, export the results to Excel and call it a day?”
My answer? “Because it won’t be scalable, robust or maintainable.” What I’m really saying is that the “secret sauce” for a world-class solar asset performance management platform has as much to do with the subject matter experts that design it as it does with the power of the performance models under the hood.
Don’t get me wrong, there is a lot of cool technology going on under the hood in Drive Pro, Power Factors’ asset performance management (APM) software platform. But if Drive Pro’s models, algorithms and methods weren’t constructed by subject matter experts — people who have gotten their hands dirty operating, maintaining and analyzing actual solar power plants — all of the shiny objects under the hood won’t be worth much.
SUMMARY
The challenge of applying the right performance model in the right place at the right time prevents many solar monitoring software platform from realizing their full potential. Until this fundamental design problem is resolved, users will continue to be frustrated with software monitoring tools that don’t work.
Want to learn more about how Power Factors’ Drive Pro asset performance management (APM) platform helps you overcome the challenge of selecting the right performance model?
Steve Hanawalt is EVP and Founder at Power Factors.