The Challenge of Imperfect Data
This is the first article in an eight-part series about the top four challenges in solar performance monitoring and how to overcome these challenges.
In this eight-part series on solar performance monitoring, we spend the first half of the series exploring four common challenges unique to solar that cause most solar asset monitoring software platforms to fail. In the second half of the series, we talk about how we can overcome these challenges once and for all.
In this first article, let’s address a foundational problem with solar asset monitoring applications: the challenge of imperfect operating data.
Defining the Challenge of Imperfect Operating Data
The purpose of a solar monitoring system is to characterize the operational performance of the plant’s operating equipment so we can ensure the equipment is performing well and in a way that is consistent with the financing model.
Model developers attempt to solve this problem by creating physical, statistical or algorithm-based models of the equipment to estimate how the equipment should perform given the actual operating conditions of the plant. Monitoring systems then feed these equipment performance models with actual operating data from the plant sensors, attempting to use the models to calculate how they can expect the equipment to perform under real-world operating conditions.
Therein lies the problem. The introduction of high volumes of imperfect, “noisy” plant operating data into the model often causes these theoretical performance models to fail. These models — which worked in the lab — return inaccurate results when faced with real-world operating data. “Inaccurate” results can mean no results at all or it can mean results that have low statistical confidence and thus should be ignored.
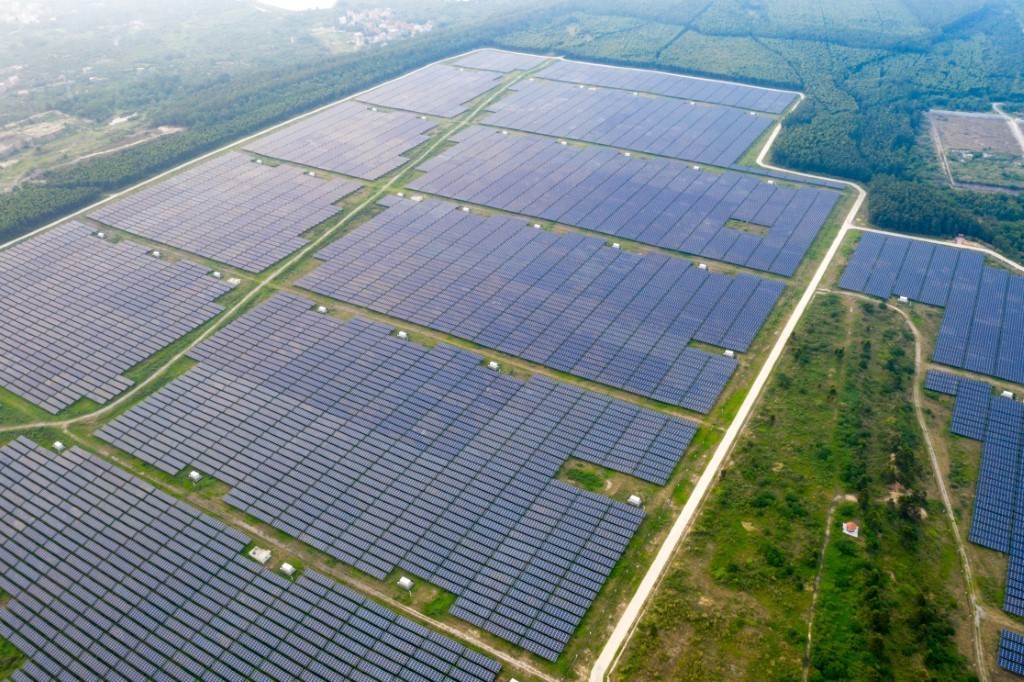
Dealing with Real-World Data
So, what are we to do? Should we just throw up our hands in defeat because the problem is too difficult to solve? No. We can’t let imperfect operating data keep us from reliable and accurate performance monitoring of our solar power fleets. The stakes are too high. Running a power plant without accurate performance data is like driving a car with no dashboard — you can do it for a while, but eventually you’re going to overheat your engine or wind up with no gas in the middle of nowhere.
And the stakes are high: In the absence of a detailed analysis of where plant performance losses are occurring, we estimate that the typical owner is forgoing 2-5% of recoverable energy production per year. For a 1 GW portfolio with an average PPA rate of $0.05/kWh, that translates to about $6M USD of additional positive cash flow for the portfolio per year.
So, what should we be doing differently to go after this incremental portfolio performance gain? We think the answer lies in the old adage, “You need to play with the cards you’ve been dealt.” Since the cost of delivering laboratory-quality data to our data models is too high, we need to let go of the dream of perfect data and deal with the messy data we have. Our performance models need to work well despite the noisy plant operating data that comes from our solar power facilities.
Is this possible? Can we develop performance models that can work with the good, the bad and the ugly of our real-world operating data? We believe the answer is “yes.” But to do this, we need to think differently about how performance monitoring systems need to work with the solar power asset class. Solar power performance models need to be resilient — and they need to inform their users of what they are capable of reliably identifying in solar plant performance shortfalls.
Summary
The problem of imperfect operating data has hampered good solar performance analysis throughout the history of the industry. Until this core problem is resolved, users will continue to be frustrated with spotty and sometimes misleading results from their monitoring tools.
Want to learn more about how Power Factors’ Drive Pro asset performance management (APM) platform helps you overcome the challenge of imperfect data?
Steve Hanawalt is EVP and Founder at Power Factors.